Urban nature and neurological health
Journal Artical
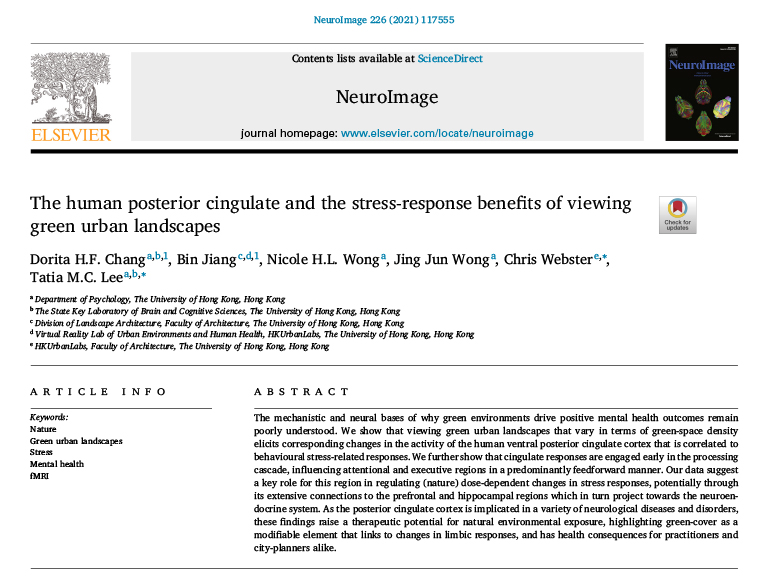
The Human Posterior Cingulate and the Stress-response Benefits of Viewing Green Urban Landscapes
NeuroImage, 2020, IF= 7.02
The mechanistic and neural bases of why green environments drive positive mental health outcomes remain poorly understood. We show that viewing green urban landscapes that vary in terms of green-space density elicits corresponding changes in the activity of the human ventral posterior cingulate cortex that is correlated to behavioural stress-related responses. We further show that cingulate responses are engaged early in the processing cascade, influencing attentional and executive regions in a predominantly feedforward manner. Our data suggest a key role for this region in regulating (nature) dose-dependent changes in stress responses, potentially through its extensive connections to the prefrontal and hippocampal regions which in turn project towards the neuroen- docrine system. As the posterior cingulate cortex is implicated in a variety of neurological diseases and disorders, these findings raise a therapeutic potential for natural environmental exposure, highlighting green-cover as a modifiable element that links to changes in limbic responses, and has health consequences for practitioners and city-planners alike.
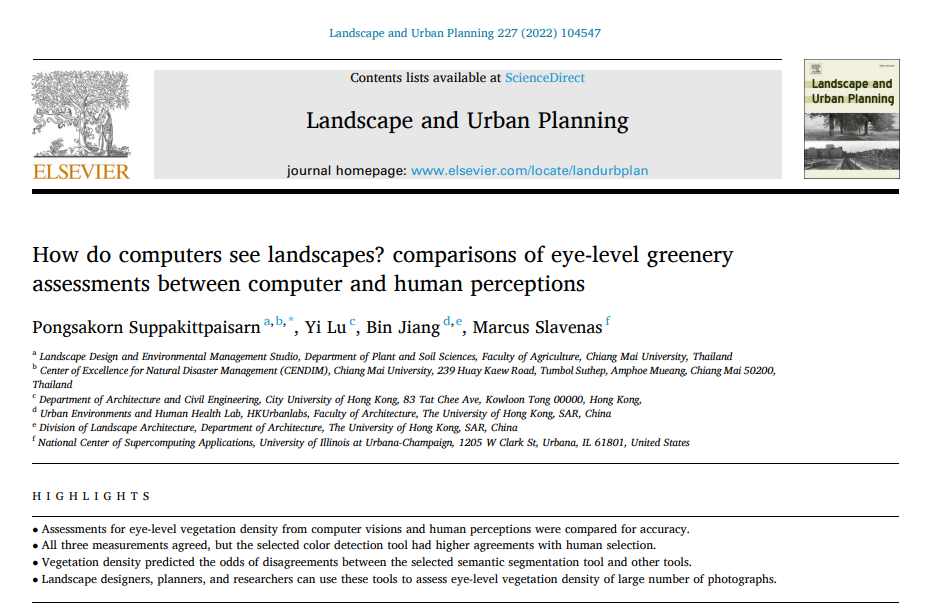
How Do Computers See Landscapes? Comparisons of Eye-level Greenery Assessments Between Computer and Human Perceptions
Landscape and Urban Planning, 2022, IF= 8.119
Landscape architects and planners have been assessing eye-level vegetation to develop evidence-based designs, including the relationships between urban nature and human health. Measuring eye-level vegetation was often subjective and time-consuming in the past. Recent advances in computer vision have made it feasible to automatically measure eye-level greenery at a large scale. However, researchers still know little about the agreements of recent machine-based methods with human perception. The research gap may lead to inaccurate or even misleading findings that may prevent effective design and planning.
This study tested the agreements between eye-level greenery detected by two machine-based methods (Brown Dog Green Index Extractor (BDGI) and PSP-Net) and human perception (manual selection via Photoshop Histogram). These two machine-based tools were selected because of their distinctive mechanisms: color detection and semantic segmentation. Cronbach’s alpha, correlation test, and Bland-Altman’s Plots were used to test agreements. Then, logistic regressions were used to find relationships between shades and vegetation density and the disagreement odds. Both tools closely agreed with human assessment in predicting eye-level greenery, with BDGI slightly closer to human. Vegetation density, but not percentage of shade, predicted the higher disagreement odds between PSP-Net and others. This finding will help advancing computer-based assessment of urban nature and contribute to our knowledge in assessing and linking eye-level greenery with potential outcomes such as physical and mental health and other design assessments.